Artificial Intelligence how can it help and harm us?
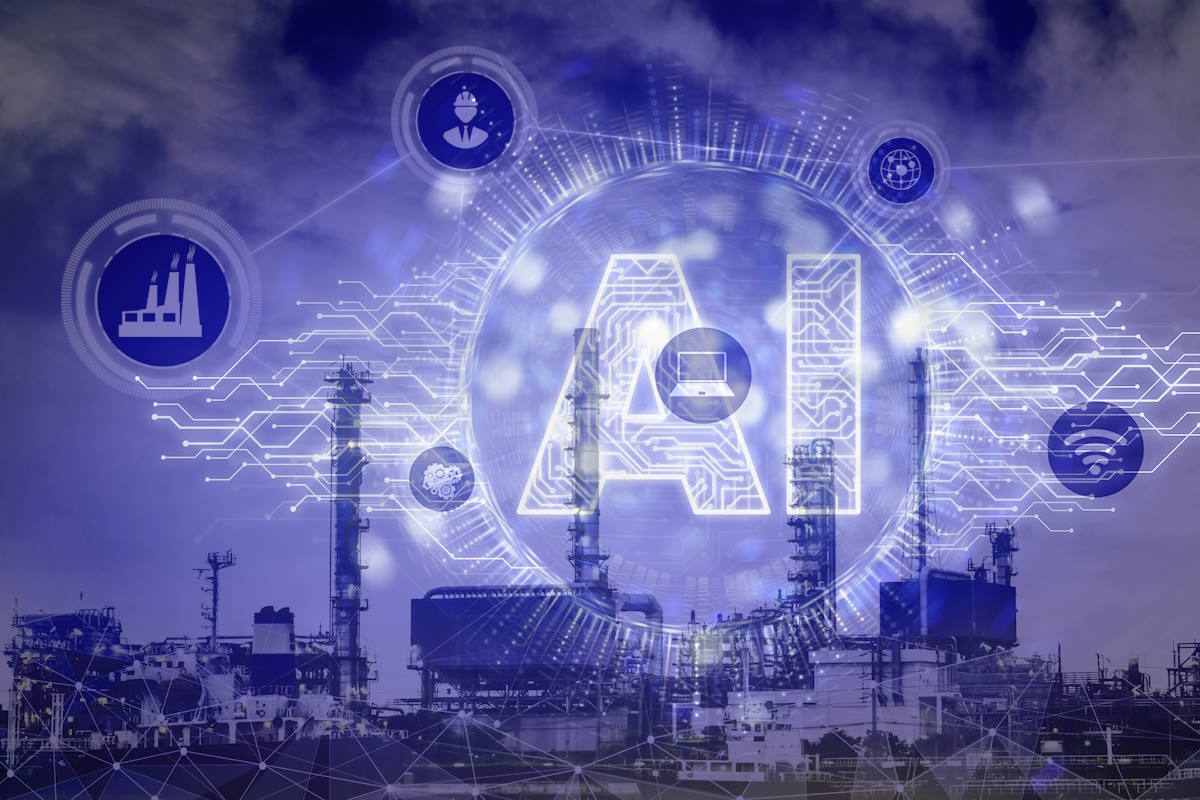
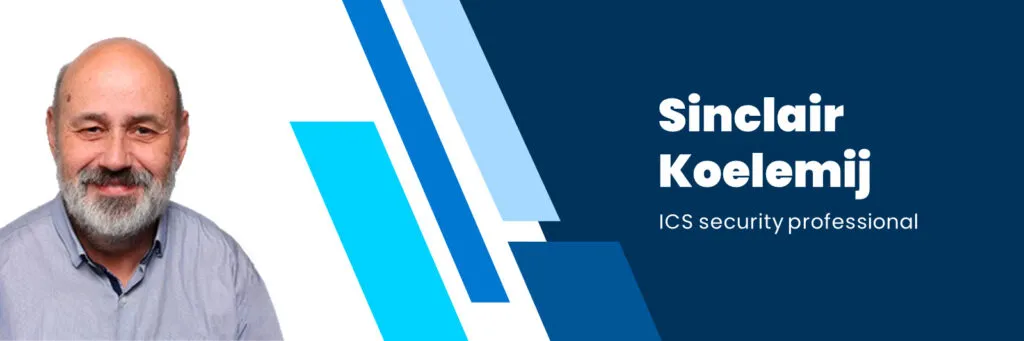
Lately, there has been a widespread discussion regarding Artificial Intelligence (AI) and its applications. Many questions have been raised about its present and future implementations, potential societal threats, and its role in bolstering cybersecurity defenses and strengthening cyber offenses. Concerns have been raised by both the industry and governments about how threat actors may exploit AI for their own gain. From all of this, it is evident that AI is a revolutionary force that is swiftly transforming both the fields of process control and OT cybersecurity, making it an important topic to discuss in this article.
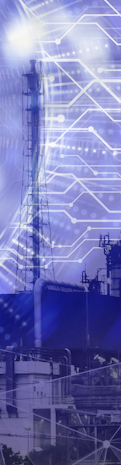
AI changes the operations, data management, and user interactions of the business, including the manufacturing industry. AI also influences the way engineers work, as engineering has always been a profession where knowing everything is not the aim. Instead, the ability to locate and apply relevant information is far more valuable. AI facilitates this by providing a structured approach to querying and responding to information, offering engineers in either camp enhanced access to valuable insights and knowledge. It is not perfect yet, so we need to be alert and challenge every result, but it certainly does contribute.
AI technology is a key component of the Industry 4.0 revolution and works in synergy with other technologies like big data and the Industrial Internet of Things (IIoT). This convergence is gradually transforming manufacturing processes, enabling organizations to harness the power of AI to improve efficiency, productivity, and decision-making. However, this integration of technologies also brings challenges, particularly in ensuring the integrity and availability of data. As data sources become more diverse and complex, it becomes increasingly difficult to trace the origin of specific data and verify its integrity and availability. This underscores the importance of implementing robust data governance and security measures to maintain the trustworthiness and reliability of AI-driven systems.
But how does AI impact the field of OT security, how can it improve cybersecurity defenses and how can it help threat actors to design better attacks? Let’s start with a short technical intro on what AI is, and how it works, and then continue how it can be applied on both the defense and offense sides.
What is AI, and how does it work?
AI systems are designed to emulate human cognitive abilities such as learning, problem solving, perception, and decision-making. These systems rely on data, collecting information to facilitate learning and using that knowledge to make predictions based on the evaluation of training data.
One approach to AI is what is called supervised learning, where the system is trained using labeled data (Closed data set). In supervised learning, the input data and corresponding output labels (the predictions) are provided, allowing the system to learn to make predictions or decisions based on the patterns and relationships found in the labeled data set.
Another approach is unsupervised learning, where the system learns from unlabeled data (uncontrolled data set) without specific input-output pairs or labels. The goal of unsupervised learning is to uncover patterns, structures, and relationships in the data without prior knowledge or guidance.
Reinforcement learning is another type of learning, where the AI system learns through trial and error based on feedback and rewards (Closed dataset). It explores different actions and learns from the outcomes, optimizing its performance over time.
The choice of learning approach has implications for the trustworthiness and subjectivity of the predictions made by the AI system. Unsupervised learning, being more exploratory in nature, can be more challenging and less controlled when the application is critical. On the other hand, supervised learning and reinforcement learning provides more control and guidance, allowing the designer to have a greater influence on the results and ensure a higher level of trust and reliability in the predictions.
Some AI techniques used are:
- Machine Learning (ML) is a subset of AI that allows systems to learn and improve from experience without explicit programming. It involves training models using datasets and utilizing statistical techniques to make predictions or take actions based on patterns and examples. These datasets can be “closed data sets” specifically created for training the AI engine, or “uncontrolled data sets” sourced from diverse sources.
- Deep Learning (DL): Deep learning is a specific type of ML that uses artificial neural networks to simulate the human brain’s structure and function. It can process complex data like images, speech, and natural language, enabling tasks such as image recognition and language translation.
- Natural Language Processing (NLP): NLP focuses on understanding and processing human language. It involves techniques like text analysis, sentiment analysis, language translation, and chatbots, allowing computers to interpret and generate human language.
- Computer Vision: Computer vision enables machines to interpret and understand visual information, such as images and videos. It involves techniques like image recognition, object detection, and image segmentation.
How can AI improve cybersecurity defense?
If we look at this question, we can refine it into topics such as:
- Can it make systems less vulnerable?
- Can it improve the detection of attacks and intrusions?
- Can it improve our response processes?
- Can it improve hazard identification and risk analysis?
Let’s have a look at each question.
How can AI make systems less vulnerable?
AI can contribute to reducing vulnerabilities and preventing cyber attacks in the following ways:
- Code Analysis: AI-powered code analysis tools can scan and analyze software code to identify coding errors, insecure practices, and potential vulnerabilities. By automatically reviewing the code, AI can assist in detecting and fixing security flaws before they can be exploited.
- Security Testing: AI-powered testing tools can simulate various types of attacks and evaluate the security of a system from different angles. These tools can uncover vulnerabilities, assess the effectiveness of security measures, and help in identifying areas that require improvement.
As AI continues to advance, it is expected that more applications will be developed to address a wider range of design factors and security considerations.
Can AI improve the detection of attacks and intrusions?
AI can enhance the detection of attacks and intrusions by leveraging its ability to analyze vast amounts of event and communication data, identify patterns, and recognize anomalies. For example:
- Anomaly Detection: AI algorithms can learn normal patterns by analyzing historical data. AI then compares real-time data against these learned patterns and flag any deviations as potential anomalies. Such as unusual network traffic, or unexpected system activities that may indicate a cyber attack.
- Behavior-based Analysis: AI can analyze user behavior and establish baselines for normal activities. It can then detect any deviations from these patterns that may indicating unauthorized access or malicious actions. Such as unusual login patterns, privilege escalation attempts, or abnormal data access patterns.
- Deep Packet Inspection: AI can perform deep packet inspection to analyze network traffic at a granular level. By examining the content and context of network packets, AI algorithms can identify potential threats, such as malicious payloads or command-and-control communications.
- User Behavior Analytics: AI can analyze user behavior across multiple systems and applications to establish a baseline of normal behavior. It can then detect any unusual activities or deviations from the baseline that may indicate a compromised user account or insider threat.
Can AI improve our response processes?
Also, for incident handling and response AI can also improve the processes by enhancing their speed, accuracy, and efficiency. Some examples are:
- Incident Triage and Prioritization: AI can analyze the severity, impact, and context of security incidents, helping security teams prioritize their response efforts. By considering various factors, such as the criticality of affected systems, potential business impact, and the likelihood of successful exploitation, AI can assist in making informed decisions on how to allocate resources effectively.
- Incident Investigation and Forensics: AI can assist in conducting efficient and thorough investigations of security incidents. It can analyze different data sources, including system logs, network traffic, and user activities, to reconstruct the attack timeline, identify the attack vectors, and determine the extent of the compromise. AI-powered analytics can accelerate the investigation process and provide valuable insights for containment and remediation.
AI can help streamline and strengthen cyber security response processes by automating tasks, providing real-time insights, continuously learning from new threats, and extending the capabilities of human analysts. It enables faster detection, response, identification, containment, and recovery, ultimately enhancing the overall resilience of the plant against cyber threats.
Can AI improve hazard identification and risk analysis?
AI can improve cyber security hazard and consequence identification, as well as enhance risk analysis for cyber-physical systems. For example:
- Data Analysis and Pattern Recognition: AI algorithms can analyze extensive data sets, including safety hazard and operability (HAZOP) documentation from many production processes, to identify potential cyber causes associated with process safety hazards and consequences. By applying advanced analytics and pattern recognition techniques it becomes possible to identify cyber-related factors that contribute to potential risks and their potential impacts on the cyber-physical system.
- Natural Language Processing (NLP) and Text Analysis: AI techniques, such as NLP and text analysis, can be utilized to analyze textual data, such as cyber and process safety incident reports, security advisories, and regulatory guidelines. By extracting relevant information and identifying key risk factors, AI can assist in hazard identification, consequence assessment, and risk analysis for cyber-physical systems.
- Machine Learning and Data-driven Insights: AI can leverage machine learning algorithms to analyze historical process and event data and identify hidden patterns or trends that may indicate potential hazards or consequences. By learning from past incidents or near-misses, AI can provide data-driven insights and predictions to enhance risk analysis and improve proactive risk mitigation strategies.
- Decision Support Systems: AI can provide decision support by integrating hazard and consequence data, risk analysis results (the risk register), and contextual information. Through analytic techniques, AI can assist engineers and process operators in understanding and recognizing complex risk scenarios, making informed decisions, and prioritizing risk mitigation actions.
So, we have ways to apply AI in many ways, some exist today, others will be developed over time. From my perspective I think AI can strengthen our defense in many ways. A proper Deep Defense strategy should apply AI. Let’s now shift our position from the blue team to the red team. How can threat actors make use of AI?
How can AI assist the threat actor?
If we look at this question, we can refine it into topics such as:
- How can it assist the reconnaissance prior to a cyber attack?
- How can it assist in cyber defense evasion?
- How can it assist in getting initial access to the system?
- How can it determine the system’s most vulnerable attack surface?
Let’s check each of the above questions.
How can AI assist the reconnaissance prior to a cyber attack?
- Automated Data Gathering: AI algorithms can automate the collection of large amounts of data from various sources, including public records, social media, and network scanning. This allows threat actors to gather information about the target organization’s infrastructure, systems, employees, and vulnerabilities.
- Social Engineering and Phishing: AI can be used to analyze social media profiles, online behavior, and communication patterns of individuals within the target organization. This information can then be used to construct targeted and convincing phishing emails or social engineering attacks.
- Automated Vulnerability Scanning: AI-powered tools can scan the target’s network and systems to identify potential vulnerabilities. These tools can automatically detect and assess vulnerabilities, reducing the time and effort required for manual reconnaissance.
- Predictive Analytics: AI algorithms can analyze historical data, including data on safety accidents, cyber attacks, and breaches, to predict potential weaknesses and vulnerabilities in the target’s infrastructure and process installation. This helps threat actors prioritize their reconnaissance efforts and focus on areas with higher chances of success.
How can AI assist cyber defense evasion?
AI can play a role in obfuscation and polymorphism by enhancing these techniques. For example:
- Obfuscation: AI can be used to develop advanced obfuscation techniques that make it difficult for defenders to understand or reverse-engineer the code. Machine learning algorithms can analyze code patterns and automatically generate obfuscated versions that preserve functionality while increasing the complexity and obscuring the original intent. AI can also help in optimizing the obfuscation process, finding the best combinations of transformations to maximize the effectiveness of obfuscated code.
- Polymorphism: AI can be used to create polymorphic malware that dynamically alters its code structure and behavior to evade detection. Machine learning algorithms can learn from the analysis of existing malware samples and generate variations that have different code structures or obfuscated patterns. This makes it harder for signature-based detection systems to identify and block polymorphic malware. AI can also assist in developing algorithms that dynamically adapt the malware’s behavior based on its environment, further enhancing its ability to evade detection.
- Adversarial Machine Learning: AI can be used to develop adversarial machine learning models that can generate inputs specifically designed to evade behavioral analysis systems. By training AI models to generate inputs that are similar to benign ones but behave differently, attackers can create samples that are less likely to trigger behavioral analysis alerts. These adversarial samples can be used to bypass or deceive the behavioral analysis algorithms.
- Anomaly Generation: AI can be employed to generate sophisticated and realistic anomalies that mimic legitimate user behaviors. By training AI models on large datasets of normal user behavior, attackers can generate anomalies that closely resemble normal patterns. These anomalies may be designed to bypass behavioral analysis systems that rely on detecting deviations from expected behavior.
- Stealthy Malware Behavior: AI can assist in the development of stealthy malware that can modify its behavior dynamically to avoid detection. AI algorithms can learn from the analysis of behavioral patterns and develop evasion strategies to mimic normal user behavior or camouflage malicious activities as legitimate ones. This makes it more challenging for behavioral analysis systems to identify and flag suspicious activities.
- Timing Obfuscation: AI can be used to dynamically adjust the timing of malicious activities to evade time-based detection mechanisms. By incorporating AI algorithms into malware or attack tools, attackers can introduce variations in the timing of their actions, making it more difficult for defenders to detect and attribute the attacks based on specific time patterns.
How can AI assist in getting initial access to the control system?
- Vulnerability Assessment: AI can assist in scanning systems and applications for usual vulnerabilities that could be exploited for initial access. By automating vulnerability assessments and leveraging AI algorithms to prioritize and analyze findings, threat actors can identify potential entry points to exploit.
- Threat Intelligence Analysis: AI can analyze vast amounts of threat intelligence data, including published indicators of compromise (IOCs) and known attack techniques, to potentially develop initial access techniques that avoid well-known paths.
- Code generation: An AI engine can be trained to generate program code based on a given specification and objective. This falls under the field of automated code generation or code synthesis. By training the AI engine on a large dataset of code samples and their corresponding specifications, it can learn the patterns, rules, and structures required to generate code that meets the given objectives. Allowing the reuse of code.
- Reverse Engineering: An AI engine can be used in the process of reverse engineering a program source based on an executable. Reverse engineering involves analyzing the binary code of an executable to understand its functionality, structure, and behavior. AI techniques, such as machine learning and pattern recognition, can aid in this process by analyzing the binary code and extracting meaningful information from it. For example, AI algorithms can be trained on a dataset of known executable files and their corresponding source code to learn patterns and relationships between the two. This trained AI model can then be used to infer the original source code based on the analysis of an unknown executable reusing malicious code.
How can AI determine the system’s most vulnerable attack surface?
- Vulnerability scanning: The AI engine can perform automated vulnerability scanning to identify weaknesses in the ICS environment. It can scan network devices, servers, and software applications to detect known vulnerabilities.
- Computer and Network Asset Discovery: The AI engine can analyze network traffic and system logs to identify and categorize ICS assets. Using machine learning algorithms, it can detect patterns and classify assets based on their unique characteristics. The AI engine can also compare this data with industry standards and vendor specifications to determine how a typical system of the vendor is configured and aligns with recommended best practices. This helps in identifying any deviations or vulnerabilities that may exist within the assets and enables organizations to take appropriate actions to address them.
- Risk assessment: The AI engine can analyze the identified assets and assess their role in the production process. It can consider factors such as the asset’s criticality, exposure to threats, and typically applied security controls to determine its vulnerability.
- Threat intelligence integration: The AI engine can integrate with threat intelligence feeds and databases to gather information on emerging vulnerabilities and threats. It can leverage this data to prioritize the potential targets and identify assets that are at higher risk.
From the above list of options, it is clear that AI offers numerous possibilities for both offensive and defensive purposes. However, as we are still in the initial stages of AI evolution, it is essential to conduct a thorough analysis of its potential applications and carefully consider the associated risks. In the current global landscape, where nation-state threats are on the rise due to increased tensions, it is crucial to create an inventory of AI-supported attack and defense scenarios. This will enable us to develop effective strategies to address these scenarios and mitigate potential risks. By proactively understanding and managing the risks posed by AI, we can harness its benefits while ensuring the security and safety of our systems and infrastructure.